breeding bugs in the latent space
A beetle generator made by machine-learning thousands of #PublicDomain illustrations.
Inspired by the stream of new Machine Learning tools being developed and made accessible and how they can be used by the creative industries, I was curious to run some visual experiments with a nice source material: zoological illustrations.
Previously I ran some test with DeepDream and StyleTransfer, but after discovering the material published at Machine Learning for Artists / @ml4a_ , I decided to experiment with the Generative Adversarial Network (GAN) approach.
Creating a Dataset
Through the Biodiversity Heritage Library, I discovered the book: Biologia Centrali-Americana :zoology, botany and archaeology, hosted at archive.org, containing fantastic #PublicDomain illustrations of beetles.
Through a combination of OpenCV and ImageMagick, I managed to extract each individual illustration and generate nicely centered square images.
Training a GAN
Following the Lecture 6: Generative models [10/23/2018] The Neural Aesthetic @ ITP-NYU, Fall 2018 - [ Lecture 6: Generative models [10/23/2018] -> Training DCGAN-tensorflow (2:27:07) ] I managed to run DCGAN with my dataset and paying with different epochs and settings I got this sets of quasi-beetles.
Nice, but ugly as cockroaches
it was time to abandone DCGAN and try StyleGAN
Expected training times for the default configuration using Tesla V100 GPUs
Training StyleGAN
I set up a machine at PaperSpace with 1 GPU (According to NVIDIA’s repository, running StyleGan on 256px images takes over 14 days with 1 Tesla GPU) 😅
I trained it with 128px images and ran it for > 3 days, costing > €125.
Results were nice! but tiny.
Generating outputs
Since PaperSpace is expensive (useful but expensive), I moved to Google Colab [which has 12 hours of K80 GPU per run for free] to generate the outputs using this StyleGAN notebook.
Results were interesting and mesmerising, but 128px beetles are too small, so the project rested inside the fat IdeasForLater folder in my laptop for some months.
In parallel, I've been playing with Runway, a fantastic tool for creative experimentation with machine learning. And in late November 2019 the training feature was ready for beta-testing.
Training in HD
I loaded the beetle dataset and trained it at full 1024px, [on top of the FlickrHD model] and after 3000 steps the results were very nice.
From Runway, I saved the 1024px model and moved it to Google Colab to generated some HD outputs.
In December 2019 StyleGAN 2 was released, and I was able to load the StyleGAN (1) model into this StyleGAN2 notebook and run some experiments like "Projecting images onto the generatable manifold", which finds the closest generatable image based on any input image, and explored the Beetles vs Beatles:
model released
make your own fake beetles with RunwayML -> start
1/1 edition postcards
AI Bugs a crypto-collectibles
Since there is an infinite number of potential beetles that the GAN can generate, I tested adding a coat of artificial scarcity and post some outputs at Makersplace as digital collectibles on the blockchain... 🙄🤦♂️


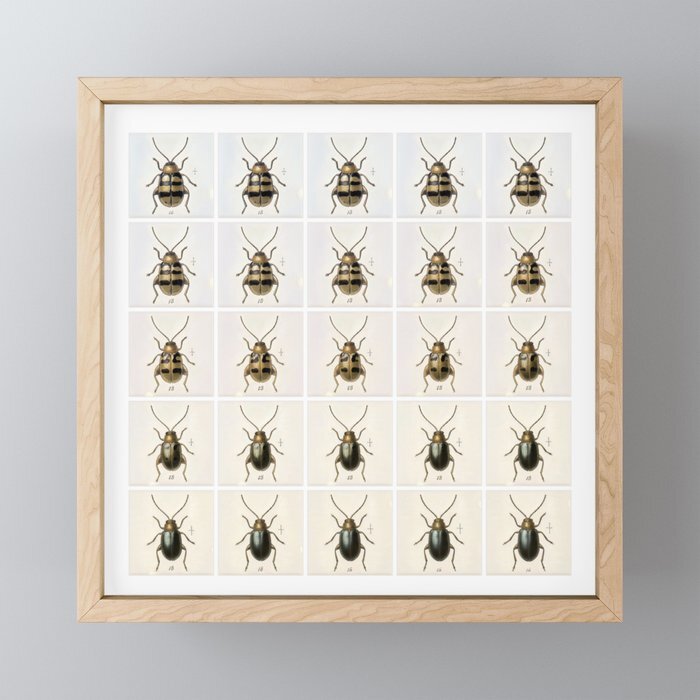
AI bugs as a prints
I then extracted the frames from the interpolation videos and created nicely organised grids that look fantastic as framed prints and posters, and made them available via Society6.
Framed prints available in six sizes, in a white or black frame color.
Every product is made just for you
Natural white, matte, 100% cotton rag, acid and lignin-free archival paper
Gesso coating for rich color and smooth finish
Premium shatterproof acrylic cover
Frame dimensions: 1.06" (W) x 0.625" (D)
Wire or sawtooth hanger included depending on size (does not include hanging hardware)
random
mini print
each print is unique [framed]