-
Notifications
You must be signed in to change notification settings - Fork 525
Commit
This commit does not belong to any branch on this repository, and may belong to a fork outside of the repository.
- Loading branch information
Showing
1 changed file
with
49 additions
and
166 deletions.
There are no files selected for viewing
This file contains bidirectional Unicode text that may be interpreted or compiled differently than what appears below. To review, open the file in an editor that reveals hidden Unicode characters.
Learn more about bidirectional Unicode characters
Original file line number | Diff line number | Diff line change |
---|---|---|
@@ -1,5 +1,4 @@ | ||
# Chatlaw: A Large Language Model-based Multi-Agent Legal Assistant Enhanced by Knowledge Graph and Mixture-of-Experts. | ||
中文法律大模型 | ||
|
||
<div align="center"> | ||
<a href="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/PKU-YuanGroup/ChatLaw"> | ||
|
@@ -20,216 +19,103 @@ | |
<img alt="GitHub stars" src="https://img.shields.io/github/stars/PKU-YuanGroup/ChatLaw?color=ccf" /> | ||
</a> | ||
<br/> | ||
<em>易用 / 简单 / 快速 </em> | ||
<br/> | ||
<a href="https://arxiv.org/pdf/2306.16092.pdf"><strong>arXiv</strong></a> | ||
· | ||
<a href="https://chatlaw.cloud/lawchat/"><strong>在线体验</strong></a> | ||
· | ||
<a href="https://huggingface.co/JessyTsu1/ChatLaw-13B"><strong>HuggingFace</strong></a> | ||
<a href="https://huggingface.co/PandaVT/ChatLaw2-Moe"><strong>HuggingFace</strong></a> | ||
</p> | ||
</p> | ||
</div> | ||
|
||
# ChatLaw系列模型 | ||
|
||
- [ChatLaw-13B](https://huggingface.co/JessyTsu1/ChatLaw-13B),此版本为学术demo版,基于姜子牙[Ziya-LLaMA-13B-v1](https://huggingface.co/IDEA-CCNL/Ziya-LLaMA-13B-v1)训练而来,中文各项表现很好,但是逻辑复杂的法律问答效果不佳,需要用更大参数的模型来解决。 | ||
|
||
- [ChatLaw-33B](https://huggingface.co/JessyTsu1/ChatLaw-33B),此版本为学术demo版,基于[Anima-33B](https://github.com/lyogavin/Anima)训练而来,逻辑推理能力大幅提升,但是因为Anima的中文语料过少,导致问答时常会出现英文数据。 | ||
|
||
- [ChatLaw-Text2Vec](https://huggingface.co/chestnutlzj/ChatLaw-Text2Vec),使用93w条判决案例做成的数据集基于BERT训练了一个相似度匹配模型,可将用户提问信息和对应的法条相匹配,例如: | ||
|
||
> “请问如果借款没还怎么办。” | ||
> | ||
> "合同法(1999-03-15): 第二百零六条 借款人应当按照约定的期限返还借款。对借款期限没有约定或者约定不明确,依照本法第六十一条的规定仍不能确定的,借款人可以随时返还;贷款人可以催告借款人在合理期限内返还。" | ||
> | ||
> 两段文本的相似度计算为0.9960 | ||
|
||
## 简介 Brief Introduction | ||
|
||
<div align="center"><img src="https://raw.githubusercontent.com/PKU-YuanGroup/ChatLaw/main/images/ChatLaw_framework.png" style="width=40%;"/></div> | ||
# ChatLaw Models | ||
|
||
ChatGPT浪潮下,人工智能的不断扩展和发展为LLM的扩散提供了肥沃的土壤,目前医疗、教育、金融领域已逐渐有了各自的模型,但法律领域迟迟没有明显进展。 | ||
## ChatLaw2-MoE | ||
|
||
为了促进LLM在法律甚至其他垂直应用落地的开放研究,本项目开源了中文法律大模型,并针对LLM和知识库的结合问题给出了法律场景下合理的解决方案。 | ||
- **Latest Version**: Based on the InternLM architecture with a 4x7B Mixture of Experts (MoE) design. | ||
|
||
ChatLaw法律大模型目前开源的仅供学术参考的版本底座为姜子牙-13B、Anima-33B,我们使用大量法律新闻、法律论坛、法条、司法解释、法律咨询、法考题、判决文书等原始文本来构造对话数据。 | ||
- **Specialization**: Tailored for Chinese legal language processing、 | ||
|
||
基于姜子牙-13B的模型是第一版模型,得益于姜子牙的优秀中文能力和我们对数据清洗、数据增强过程的严格要求,我们在逻辑简单的法律任务上表现优异,但涉及到复杂逻辑的法律推理任务时往往表现不佳。 | ||
## ChatLaw-13B | ||
|
||
随后基于[Anima-33B](https://github.com/lyogavin/Anima),我们增加了训练数据,做成了ChatLaw-33B,发现逻辑推理能力大幅提升,由此可见,大参数的中文LLM是至关重要的。 | ||
- **Demo Version**: Built on the Ziya-LLaMA-13B-v1 model. | ||
- **Performance**: Excels in general Chinese tasks but requires a larger model for complex legal QA. | ||
|
||
我们的技术报告在这里: [arXiv: ChatLaw](https://arxiv.org/pdf/2306.16092.pdf) | ||
## ChatLaw-33B | ||
|
||
基于可商用的模型训练而成的版本会作为我们后续产品内部接入的版本,对外不开源,可以在[这里](https://chatlaw.cloud/lawchat/)进行开源版本模型的试用 | ||
- **Demo Version**: Utilizes the Anima-33B model. | ||
- **Enhancements**: Improved logical reasoning over the 13B version. | ||
- **Challenge**: Occasionally defaults to English responses due to limited Chinese training data in Anima. | ||
|
||
## ChatLaw-Text2Vec | ||
|
||
## 数据 Dataset | ||
- **Function**: A text similarity model trained on 93,000 court case decisions. | ||
- **Capability**: Matches user queries to pertinent legal statutes, offering contextual relevance. | ||
- **Example**: Connects questions about loan repayment to the appropriate sections of contract law. | ||
|
||
我们的数据主要由论坛、新闻、法条、司法解释、法律咨询、法考题、判决文书组成,随后经过清洗、数据增强等来构造对话数据。详细的参考数据在[ChatLaw训练数据demo](https://github.com/PKU-YuanGroup/ChatLaw/tree/main/data),以下是数据样例: | ||
|
||
| question | answer | Subject | | ||
| ------------------------------------------------------------ | ------------------------------------------------------------ | ---------- | | ||
| 如果一个人故意驾车向人群冲撞,但没有造成重大损失,会被怎样处罚? | 依据刑法条文第一百一十四条,如果一个人故意以危险方法危害公共安全,但尚未造成严重后果,将被处三年以上十年以下有期徒刑。因此,如果这个人故意驾车向人群冲撞,但没有造成重大损失,将被处以三年以上十年以下有期徒刑。 | 法律咨询 | | ||
| 如果一个邮政工作人员私自开拆了一个邮件,但只是为了查看其中的收件人地址,而并未泄露邮件内容,是否构成什么罪行? | 构成私自开拆、隐匿、毁弃邮件、电报罪。本罪客观方面有两个特点:一是必须有私自开拆、隐匿、毁弃邮件、电报的行为。所谓私自开拆,是指非法擅自开拆他人邮件、电报,使封缄失效的行为,合法行为不在此限;二是必须有利用职务之便的行为,才能构成本罪。即使只是查看收件人地址也违反了邮政工作人员的职责,属于利用职务之便的行为,因此构成本罪。 | 法律概念 | | ||
| 如果某个工人每天工作10小时,那么每周工作时间超过了规定吗? | 根据《劳动法》第三十六条,每日工作时间不得超过8小时,因此每周工 作时间将超过44小时,超过了规定。 | 法律咨询 | | ||
| 甲公司注册资金为120万元,主营建材,乙厂为生产瓷砖的合伙企业。甲公司为稳定货源,决定投资30万元入伙乙厂。对此项投资的效力,下列表述哪一项是正确的?<br/>A)须经甲公司股东会全体通过方为有效<br/>B)须经甲公司董事会全体通过方为有效<br/>C)须经乙厂全体合伙人同意方为有效<br/>D)无效| 按照最新《公司法》的规定,本题无答案。《公司法》第15条规定,公司可以向其他企业投资;但是,除法律另有规定外,不得成为对所投资企业的债务承担连带责任的出资人...... | 法律选择题 | | ||
## Introduction | ||
|
||
<div align="center"><img src="https://raw.githubusercontent.com/PKU-YuanGroup/ChatLaw/chatlaw2/assert/main.jpg" style="width=40%;"/></div> | ||
|
||
AI legal assistants, powered by Large Language Models (LLMs), offer accessible legal consulting. However, the risk of hallucination in AI responses is a concern. This paper introduces ChatLaw, an innovative assistant that employs a Mixture-of-Experts (MoE) model and a multi-agent system to enhance reliability and accuracy in AI legal services. By integrating knowledge graphs and artificial screening, we've created a high-quality legal dataset for training the MoE model. This model leverages various experts to address a range of legal issues, optimizing legal response accuracy. Standardized Operating Procedures (SOPs), inspired by law firm workflows, significantly minimize errors and hallucinations. | ||
|
||
## 实验结果 experiments | ||
Our MoE model outperforms GPT-4 in the Lawbench and Unified Qualification Exam for Legal Professionals, achieving a 7.73% higher accuracy and an 11-point lead, respectively. It also surpasses other models in real-case consultations across multiple dimensions, showcasing robust legal consultation capabilities. | ||
|
||
如何合理地评估垂直领域大模型的性能一直是一个问题,因为测试数据和真实场景存在差异,我们暂时没有完美的思路。我们只是收集了十余年的国家司法考试题目,整理出了一个包含2000个问题及其标准答案的测试数据集,用以衡量模型处理法律选择题的能力: [评测数据demo](https://github.com/PKU-YuanGroup/ChatLaw/tree/main/data/ELO_val)。 | ||
--- | ||
|
||
然而,我们发现各个模型的准确率普遍偏低。在这种情况下,仅对准确率进行比较并无多大意义。因此,我们借鉴英雄联盟的ELO匹配机制,做了一个模型对抗的ELO机制,以便更有效地评估各模型处理法律选择题的能力。以下分别是ELO分数和胜率图 | ||
### Multi-Agents Collaboration Process and Legal Consultation Report | ||
|
||
<div style="text-align: center;"> | ||
<img src=https://raw.githubusercontent.com/PKU-YuanGroup/ChatLaw/main/images/elo.jpg width="200"/> | ||
<img src=https://raw.githubusercontent.com/PKU-YuanGroup/ChatLaw/main/images/win_rate.png width="500"/> | ||
</div> | ||
|
||
有以下结论:(1)引入法律相关的问答和法规条文的数据,能在一定程度上提升模型在选择题上的表现;(2)加入特定类型任务的数据进行训练,模型在该类任务上的表现会明显提升。例如,ChatLaw模型之所以能胜过GPT-4,是因为我们使用了大量选择题作为训练数据;(3)法律选择题需要进行复杂的逻辑推理,因此,参数量更大的模型通常表现更优。 | ||
|
||
## 效果 Results | ||
|
||
**注意:** 下面的测试图中加入了法条检索模块,因此会有更好的效果。**目前由于在线体验网站压力过大,服务器资源不足,我们关闭了法条检索模块**。 | ||
The diagram below illustrates the collaborative process of multiple agents in providing legal consultation services, exemplified by a divorce consultation. The process involves gathering information, legal research, comprehensive advice, and culminates in a detailed Legal Consultation Report. | ||
|
||
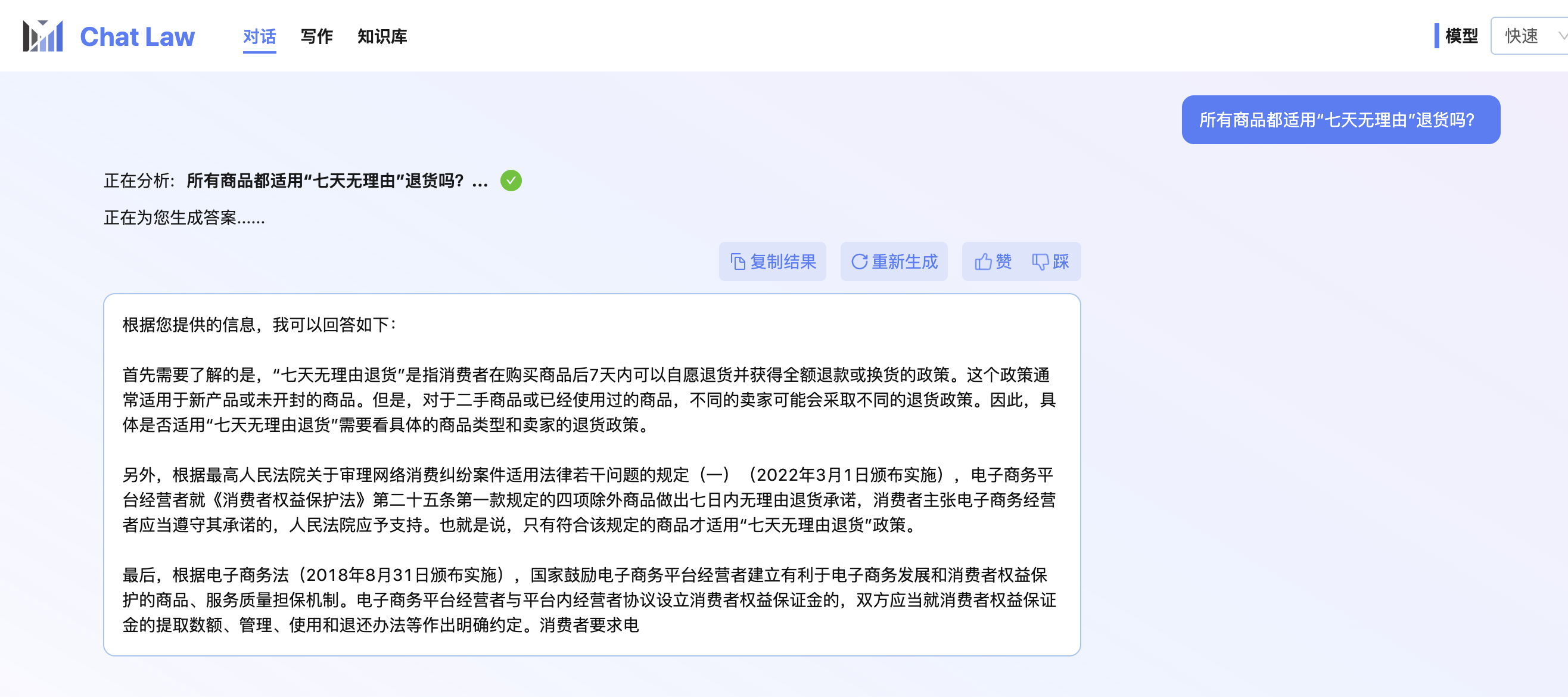 | ||
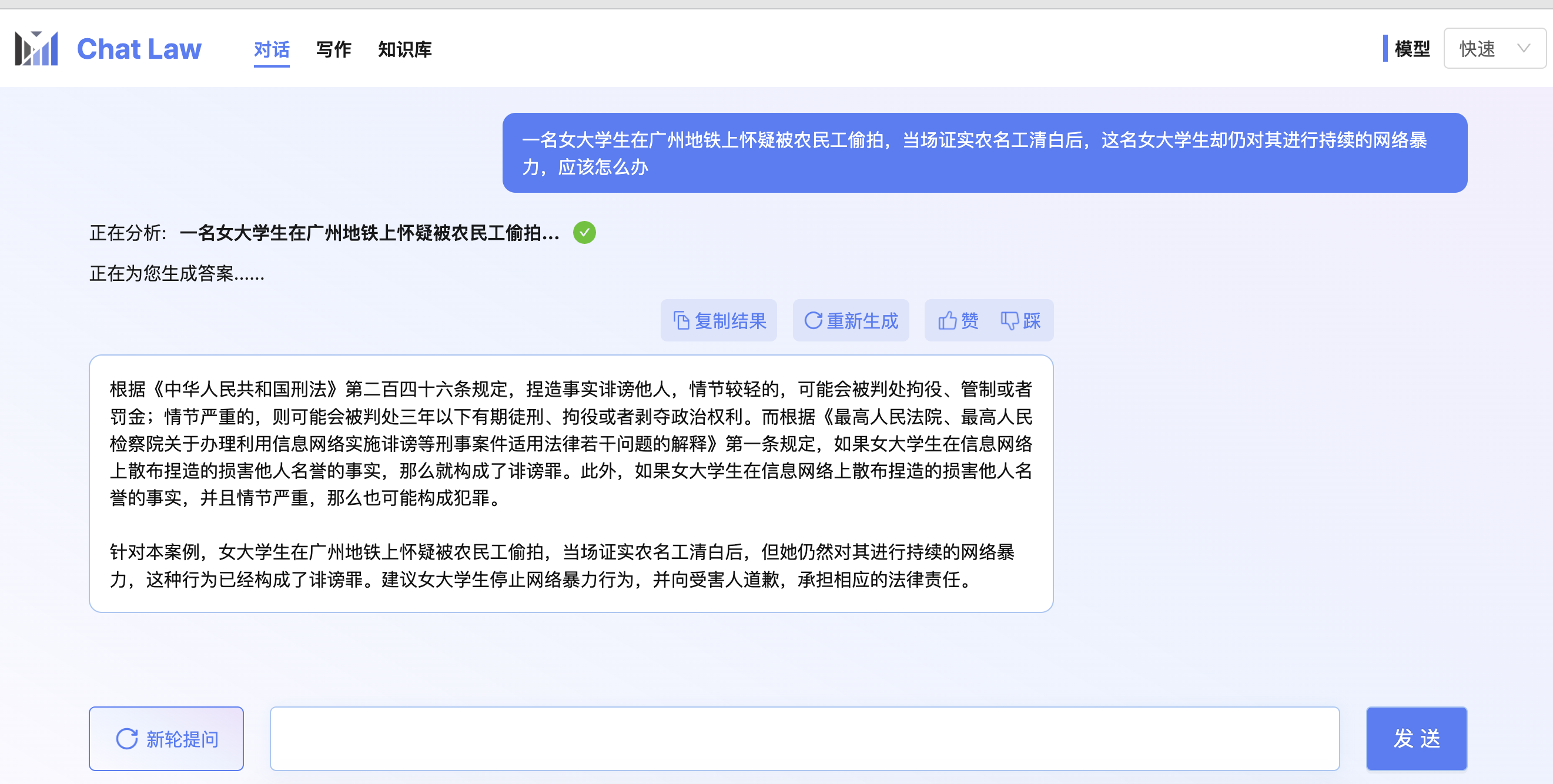 | ||
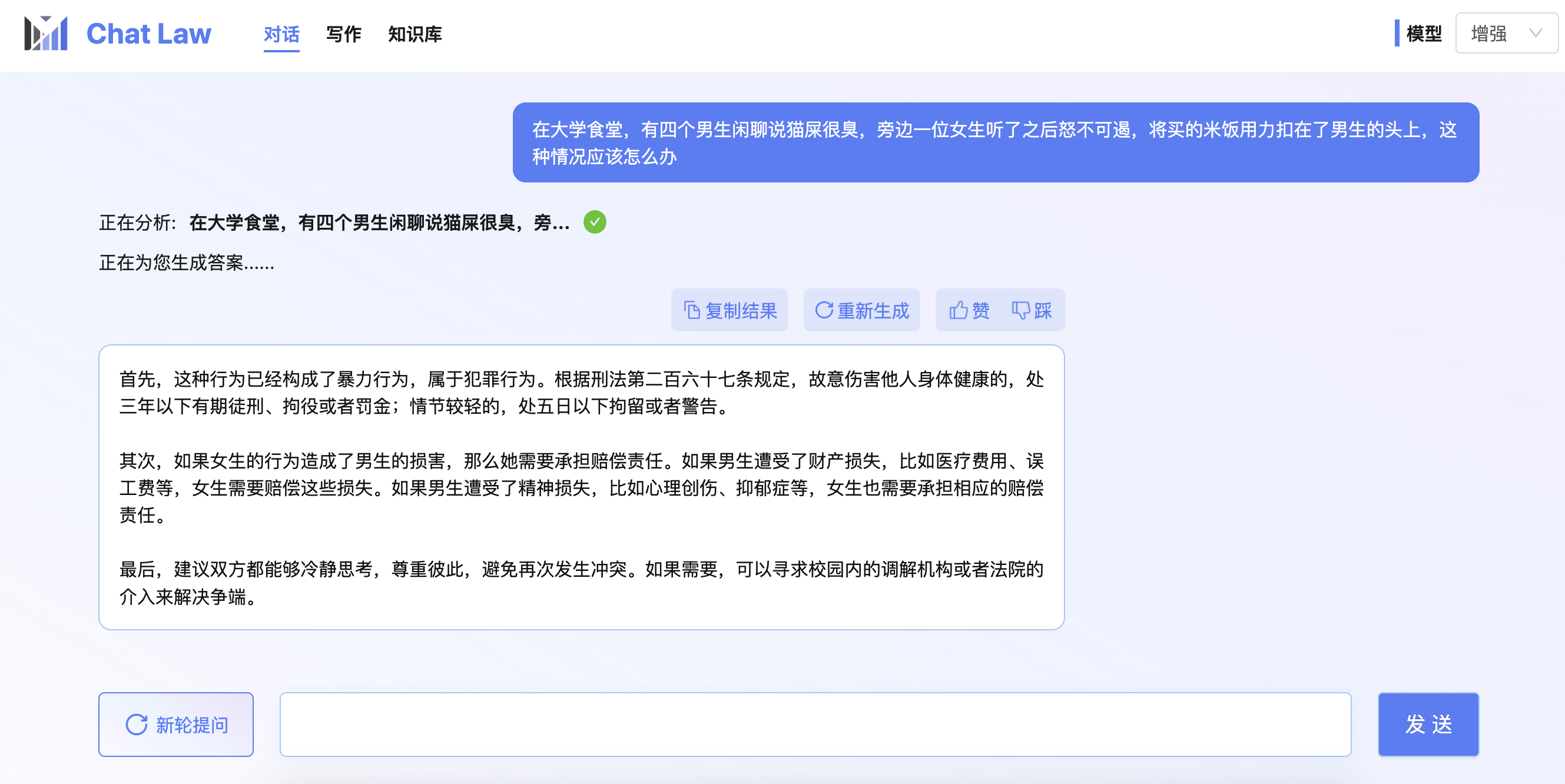 | ||
<div align="center"><img src="https://raw.githubusercontent.com/PKU-YuanGroup/ChatLaw/chatlaw2/assert/fig3.jpg" style="width=40%;"/></div> | ||
|
||
## 未来计划 | ||
# Dataset | ||
|
||
+ **提升逻辑推理能力,训练30B以上的中文模型底座**:在ChatLaw的迭代过程中,我们发现和医疗、教育、金融等垂直领域不同的是,法律场景的真实问答通常涉及很复杂的逻辑推理,这要求模型自身有很强的逻辑能力,预计只有模型参数量达到30B以上才可以。 | ||
For datasets, please visit: | ||
|
||
+ **安全可信,减少幻觉**:法律是一个严肃的场景,我们在优化模型回复内容的法条、司法解释的准确性上做了很多努力,现在的ChatLaw和向量库结合的方式还可以进一步优化。 | ||
+ **私有数据模型**:我们一方面会继续扩大模型的基础法律能力,另一方面会探索B/G端的定制化私有需求,欢迎探讨合作 | ||
- [Chinese Law Examples](https://huggingface.co/datasets/PandaVT/chinese_law_examples) | ||
- [Chinese Verdict Examples](https://huggingface.co/datasets/PandaVT/chinese_verdict_examples) | ||
|
||
本Demo只是一个开始,我们将致力于开发面向大众的普惠法律产品,期待通过大模型助力法律资源的再分配和生产力的提升,“但愿世间不纷争,何惜法典卷生尘”是我们的宗旨。 | ||
## Data Visualization and Performance Comparison | ||
|
||
如果您有进一步了解产品、进行投资、商务合作的需求,请联系[email protected] | ||
(a) Our legal dataset covers a diverse range of tasks, from case classification to public opinion analysis. | ||
|
||
如果您是法律人/技术开发者/需求者,想进一步了解团队或加入我们,欢迎添加微信13834965808,请写明来意,我们期待与您的交流。 | ||
(b) ChatLaw demonstrates superior performance across multiple legal categories compared to other models. | ||
|
||
## 项目参与者 | ||
(c) ChatLaw consistently outperforms other models in legal cognitive tasks, as shown in the Lawbench comparison. | ||
|
||
本项目由北京大学深圳信息工程学院完成,指导教师为[袁粒](https://yuanli2333.github.io/) | ||
(d) ChatLaw maintains high performance across five years on the Unified Qualification Exam for Legal Professionals. | ||
|
||
以下为相关贡献者,排名不分先后 | ||
<div align="center"><img src="https://raw.githubusercontent.com/PKU-YuanGroup/ChatLaw/chatlaw2/assert/fig1.jpg" style="width=40%;"/></div> | ||
|
||
发起人:[陈伯华](https://github.com/cbh1733908441)、[崔家熙](https://github.com/JessyTsu1) | ||
# Experiments | ||
|
||
研发:[WLJ](https://github.com/Jditbs)、[Rikka](https://github.com/qqingzheng)、[李桢](https://github.com/LZpenguin)、[熊博](https://github.com/BearBo666)、[牛天翔](https://github.com/moisoc)、[刘星宇](https://github.com/LkkkLxy)、[晏阳](https://github.com/yanyang1024)、[童维希](https://github.com/VichyTong)、[谢晗琦](https://github.com/xhq18397777970) | ||
## Evaluation of Legal Consultation Quality and Model Performance | ||
|
||
视频:[Zorn Wang](https://github.com/ZornWang) | ||
(a) Legal consultation quality is assessed based on Completeness, Logic, Correctness, Language Quality, Guidance, and Authority. | ||
|
||
UI设计:毛茜茜 | ||
(b) ChatLaw achieves the highest scores across all criteria, particularly excelling in Completeness, Guidance, and Authority. | ||
|
||
Hr:向曾 | ||
(c) ChatLaw shows a higher win rate compared to other models, indicating superior capability in providing high-quality legal consultations. | ||
|
||
## 使用 Usage | ||
<div align="center"><img src="https://raw.githubusercontent.com/PKU-YuanGroup/ChatLaw/chatlaw2/assert/fig2.jpg" style="width=40%;"/></div> | ||
|
||
由于LLaMA权重的许可限制,该模型不能用于商业用途,请严格遵守LLaMA的使用政策。考虑到LLaMA权重的许可证限制,我们无法直接发布完整的模型权重。 | ||
## Truthful QA Evaluation | ||
|
||
步骤1:获取[LLaMA](https://docs.google.com/forms/d/e/1FAIpQLSfqNECQnMkycAp2jP4Z9TFX0cGR4uf7b_fBxjY_OjhJILlKGA/viewform)原始权重并转成Hugging Face Transformers模型格式(简称为hf格式),可参考转换脚本[convert_llama_weights_to_hf.py](https://github.com/huggingface/transformers/blob/main/src/transformers/models/llama/convert_llama_weights_to_hf.py),将该代码复制粘贴保存到本地。 | ||
|
||
进入到convert_llama_weights_to_hf.py的同级目录,打开命令行执行: | ||
```bash | ||
python convert_llama_weights_to_hf.py --input_dir {原始 llama-13b 权重路径} --model_size 13B --output_dir 保存路径 | ||
``` | ||
|
||
例如 | ||
|
||
```bash | ||
python convert_llama_weights_to_hf.py --input_dir /home/llama-13b --model_size 13B --output_dir /home/llama-13b-hf | ||
``` | ||
<div align="center"><img src="https://raw.githubusercontent.com/PKU-YuanGroup/ChatLaw/chatlaw2/assert/truthfulqa.jpg" style="width=40%;"/></div> | ||
|
||
## Usage | ||
|
||
步骤2:下载[Ziya-LLaMA-13B-v1](https://huggingface.co/IDEA-CCNL/Ziya-LLaMA-13B-v1)的 delta 权重,使用如下脚本合并Ziya-LLaMA-13B-v1 的 delta 权重与 hf格式的LLaMA权重,得到完整版的Ziya-LLaMA-13B-v1模型权重。合并脚本链接:[https://github.com/IDEA-CCNL/Fengshenbang-LM/blob/main/fengshen/utils/apply_delta.py](https://github.com/IDEA-CCNL/Fengshenbang-LM/blob/main/fengshen/utils/apply_delta.py)。 | ||
Find the model at: [ChatLaw2-MoE](https://huggingface.co/PandaVT/ChatLaw2-MoE) | ||
|
||
同理,将apply_delta.py中的代码复制粘贴到本地 | ||
|
||
进入到apply_delta.py的同级目录,打开命令行执行: | ||
|
||
```bash | ||
python apply_delta.py --base {hf格式的LLaMA权重路径} --target {保存路径} --delta {Ziya-LLaMA-13B-v1 的 delta 权重路径} | ||
``` | ||
|
||
例如 | ||
|
||
```bash | ||
python3 apply_delta.py --base /home/llama-13b-hf --target /home/Ziya-LLaMA-13B --delta /home/Ziya-LLaMA-13B-v1 | ||
``` | ||
|
||
步骤3:合并ChatLaw权重并推理 | ||
|
||
```python | ||
import re | ||
import torch | ||
from peft import PeftModel | ||
from transformers import GenerationConfig, LlamaForCausalLM, LlamaTokenizer | ||
|
||
def main(): | ||
ziya_model_path = "/home/Ziya-LLaMA-13B" # 完整的子牙模型权重路径 | ||
chatlaw_model_path = "/home/chatlaw" # chatlaw模型权重 | ||
tokenizer = LlamaTokenizer.from_pretrained(ziya_model_path) | ||
model = LlamaForCausalLM.from_pretrained( | ||
ziya_model_path, | ||
torch_dtype=torch.float16, | ||
device_map="auto", | ||
) | ||
|
||
model = PeftModel.from_pretrained(model, chatlaw_model_path) | ||
|
||
if tokenizer.pad_token is None: | ||
tokenizer.pad_token = tokenizer.unk_token | ||
|
||
model.eval() | ||
|
||
consult = "介绍一下内控行业" | ||
prompt = f"Consult:\n{consult}\nResponse:\n" | ||
|
||
inputs = tokenizer(prompt, return_tensors="pt") | ||
inputs['input_ids'] = inputs['input_ids'].to(model.device) | ||
|
||
generation_config = GenerationConfig( | ||
temperature=0.1, | ||
top_p=0.75, | ||
top_k=40, | ||
num_beams=4 | ||
) | ||
|
||
with torch.no_grad(): | ||
generation_output = model.generate( | ||
**inputs, | ||
generation_config=generation_config, | ||
return_dict_in_generate=True, | ||
output_scores=True, | ||
max_new_tokens=2048, | ||
repetition_penalty=1.2, | ||
) | ||
s = generation_output.sequences[0] | ||
output = tokenizer.decode(s) | ||
if search_result := re.search("Response\s*:\s*([\s\S]+?)</s>", output): | ||
output = search_result.group(1) | ||
print(output) | ||
|
||
if __name__ == "__main__": | ||
main() | ||
``` | ||
|
||
|
||
|
||
|
||
|
||
欢迎引用我们: | ||
|
||
``` | ||
@misc{cui2023chatlaw, | ||
title={ChatLaw: Open-Source Legal Large Language Model with Integrated External Knowledge Bases}, | ||
author={Jiaxi Cui and Zongjian Li and Yang Yan and Bohua Chen and Li Yuan}, | ||
year={2023}, | ||
@misc{cui2024chatlaw, | ||
title={Chatlaw: A Multi-Agent Collaborative Legal Assistant with Knowledge Graph Enhanced Mixture-of-Experts Large Language Model}, | ||
author={Jiaxi Cui and Munan Ning and Zongjian Li and Bohua Chen and Yang Yan and Hao Li and Bin Ling and Yonghong Tian and Li Yuan}, | ||
year={2024}, | ||
eprint={2306.16092}, | ||
archivePrefix={arXiv}, | ||
primaryClass={cs.CL} | ||
|
@@ -256,7 +142,4 @@ if __name__ == "__main__": | |
|
||
<a href="https://github.com/PKU-YuanGroup/ChatLaw/graphs/contributors"> | ||
<img src="https://contrib.rocks/image?repo=PKU-YuanGroup/ChatLaw" /> | ||
</a> | ||
|
||
|
||
|
||
</a> |